Examining Model Sensitivity Across Scales
Running large-eddy simulations (LESs) for real-data cases is often computationally expensive. Thus, it is common – in practice – to run an ensemble of mesoscale simulations in order to find the best numerical setup to drive a single LES. But can we in fact assume that model performance or model sensitivity to certain parameters or inputs is constant across scales? Will the best model setup on the mesoscale translate to the best microscale performer?
In this study, sea surface temperature (SST) is augmented in an ensemble of mesoscale-to-microscale simulations for an offshore low-level jet (LLJ) case study in order to determine if model sensitivity is consistent across scales. LLJ characteristics and near-surface stability is impacted by differences in the low-level temperature and SST. The various SST datasets include different satellite and in-situ observations and are output at varying grid spacing. This impacts how well SST gradients and ocean features are resolved which, in turn, impacts the low-level stability and LLJ characteristics.
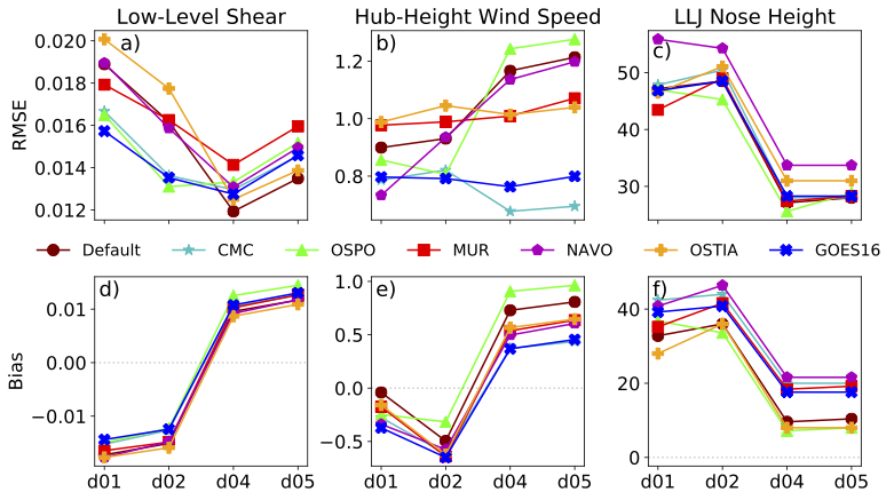
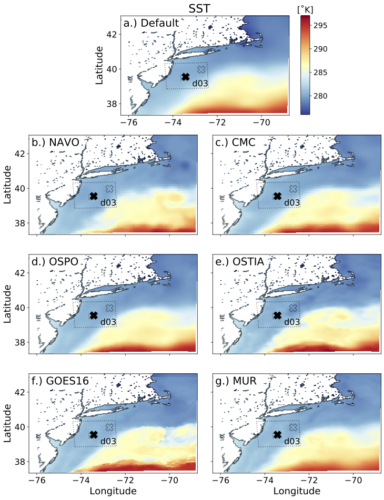
We find that model bias and error are not constant across scales which causes the best mesoscale performers to not necessarily become the best microscale performers. For example, the OSPO dataset is one of the better mesoscale performers, but becomes one of the worst performers when considering the microscale domains. This difference is thought to be caused by inherent differences in the mesoscale and microscale parameterizations of unresolved turbulence.